Redefining AI Conversations: How Retrieval Augmented Generation is supercharging Large Language Models for a smarter future.
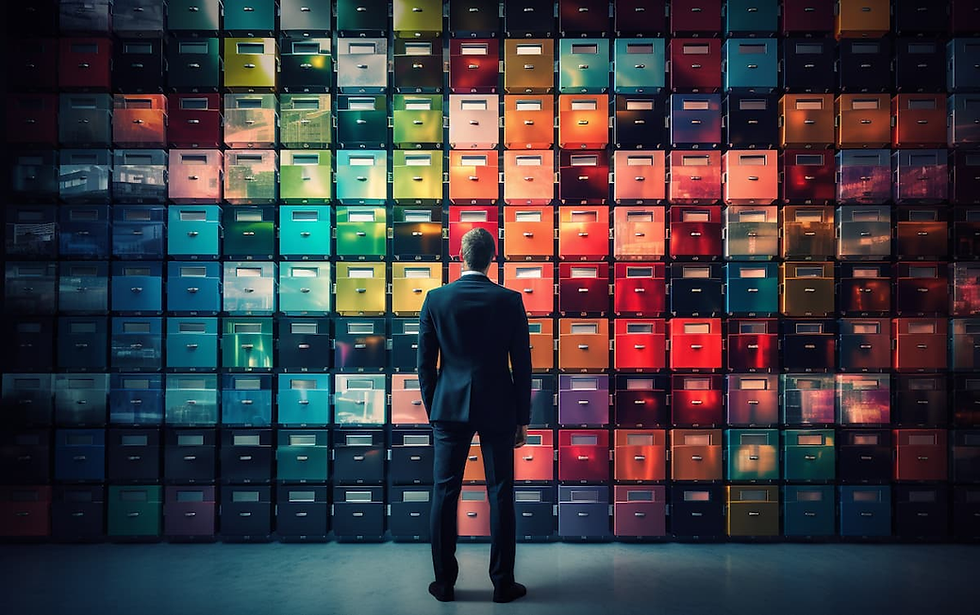
The Generative AI Revolution: An Introduction to Retrieval Augmented Generation
The release of ChatGPT in November 2022 sparked tremendous excitement about the potential for large language models (LLMs) like it to revolutionize how people and organizations use AI. However, in their default form, these models have limitations around working with custom data.
This is where the idea of retrieval augmented generation (RAG) comes in. RAG is a straightforward technique that enables LLMs to dynamically incorporate external context from databases. By retrieving and appending relevant data to prompts, RAG allows LLMs to produce high-quality outputs personalized to users' needs.
Since ChatGPT burst onto the scene, interest in RAG has skyrocketed. Organizations want to leverage powerful models like ChatGPT while also equipping them with proprietary company data and knowledge. RAG offers a way to get the best of both worlds.
In this article, we will provide an overview of RAG and its applications. While the core principle is simple, RAG unlocks a breadth of new possibilities for generating customized, grounded content with LLMs. Join us as we explore this versatile technique fueling the next evolution of AI!
What is Retrieval Augmented Generation (RAG)? A Simple Explanation
When using a large language model (LLM) like ChatGPT, you can provide additional context by including relevant information directly in the prompt. For example, if you paste the text of a news article into the prompt, ChatGPT can use that context to generate a timeline of events.
Retrieval augmented generation (RAG) works similarly but automates this process using a vector database. Instead of manually adding information to the prompt, relevant data is automatically retrieved from the database and combined with the original prompt.
You input an initial prompt to the system, such as "Please summarize the key events in the news recently."
Behind the scenes, the system searches a vector database full of up-to-date news articles and finds relevant hits based on semantic similarity.
These retrieved articles are combined with the original prompt to create an expanded prompt with additional context.
This new "augmented" prompt looks something like this: "Please summarize the key events in the news recently. Yesterday, there was a 7.2 earthquake in Turkey. The day before, NASA launched a new spaceship..."
The expanded prompt is fed to the LLM, which now has the context to generate a useful summary grounded in real data.
Comentários